The ever-evolving landscape of machine learning: a comprehensive guide
INTRODUCTION
Machine Learning is a transformative field at the intersection of computer science and artificial intelligence, revolutionizing the ay we approach complex problems and make sense of vast amount of data. At its core, machine learning is the set of algorithms and technique that enables computer to learn and adapt from experience, rather than relying on explicit programming. This technology has unlocked remarkable possibilities in various domains, from healthcare and finance to autonomous vehicles and natural language processing, making it a driving force behind the next generation of intelligent system. As the demand for data-driven insight continues to grow, machine learning stand as a pivotal tool in harnessing the power of data to automate tasks, make predictions,and uncover hidden patterns promising a future where machines truly learn to assist, inform, and empower to us. This article delve deep into the fascinating world of machine learning, its applications, advancements, and societal impact it has been creating over the years. From self-driving cars to healthcare diagnostics, financial predictions to recommendation system, machine learning is at the heart of these innovation.
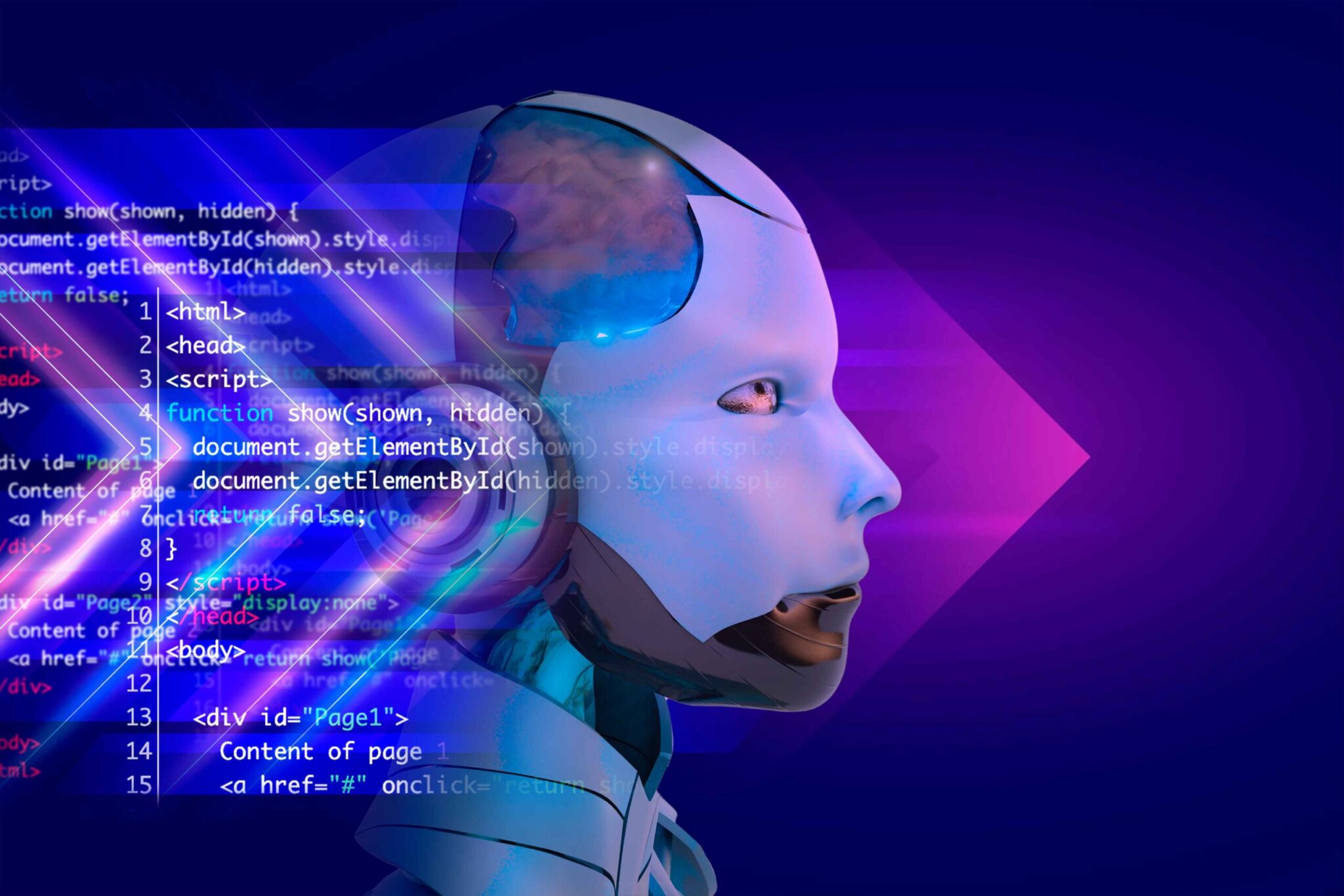
What is Machine Learning?
Machine Learning is a dynamic and rapidly evolving field within the realm of Artificial Intelligence that empowers computers to learn and improve their performance on specific tasks through data-driven experiences, rather than relying on explicit programming. This transformative technology allow machines to recognize patterns, make predictions, and autonomously adapt to new information, mirroring the way human learn. At its heart, machine learning harnesses the vast potential of data and algorithm to enhance decision-making, automate processes, and derive insights from complex information. With applications spanning a wide range of domains, from recommendation system and image recognition and predictive analytics and autonomous robotics, machine learning has emerged as a cornerstone of the modern technological landscape, poised to shape the future in profound ways.
This means that instead of giving a computer step-by-step instructions, we provide it with data, and it learn patterns and rules from that data to make predictions or decisions. This technology inspires by the way human learns, has rapidly evolved and is now integral to an array of application across numerous domains. By leveraging statistical techniques and data-driven insights, machine learning has the remarkable capability to decipher patterns, make predictions, and provide valuable insights from vast datasets, ushering in a future where computers can adapt, reasons can make informed decisions autonomously, fundamental altering the way we approach problems solving and automation.
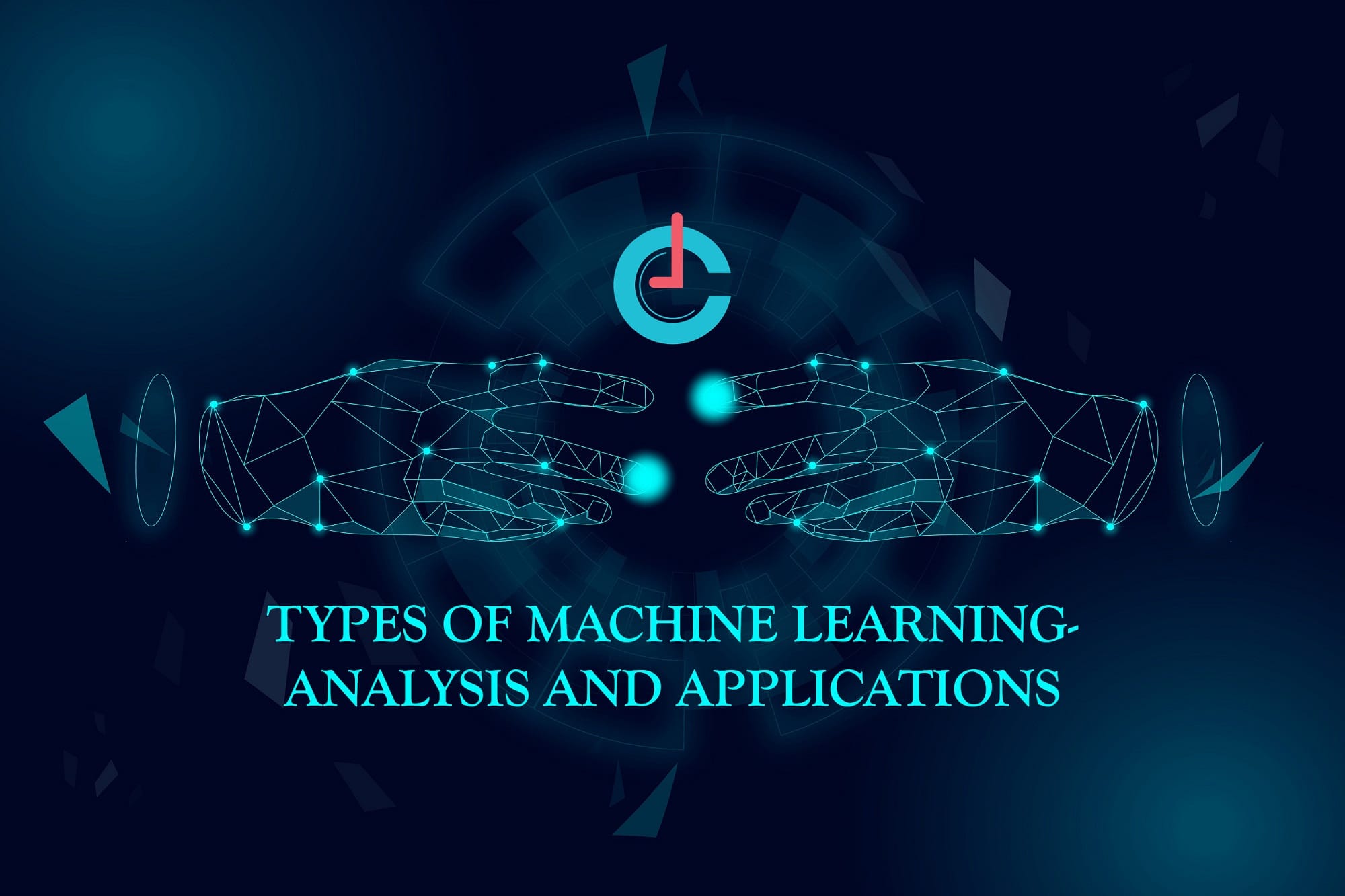
Types of Machine Learning
Machine Learning algorithms can be categorized into three main types:
Supervised Learning
Supervised Learning stand as one of the foundation pillars of machine learning, serving as a guiding light in the quest to make machines understand and interpret data. In this approach, the machine is trained on a labeled datasets, where it learns to recognize patterns and make predictions under the guidance of a teacher, or in this case, a labeled datasets. The core principle behind supervised learning is the notion of providing clear explicit examples for the models to grasp, allowing it to generalize and make predictions on new unseen data accurately. Whether its classifying images, predicting stock prices, or diagnosing medical conditions, supervised learning algorithms have proven to be invaluable tools in various fields, paving the way for data-driven decision making and automation in an ever evolving technological landscape.
Unsupervised Learning
Unsupervised Learning is a captivating realm within the field of machine learning, where the focus shifts from labeled data and guidance to the intrinsic organization and pattern hidden within unstructured information. Unlike unsupervised learning, in this approach, algorithm ventures into the uncharted territory of unlabeled data, seeking to unearth underlying structures, groupings or relationship autonomously. This method is particularly adept at tasks like clustering, dimensionality reduction, and anomaly detection, where the goal is to discover previously unknown insights and intrinsic patterns, making it a vital tool for exploratory data analysis and uncovering hidden knowledge and vast and complex datasets. Unsupervised learning open the door to a deeper understanding of data, enabling machines to extract meaning and associations from the raw, unprocessed information, a pivotal capability with application across a spectrum of disciplines and industries.
Reinforcement Learning
Reinforcement Learning is a captivative branch of machine learning, simulates the way humans sand animals learn from interactions with their environments. In this paradigm, an agent often represented by a computer program or a robot, is equipped with the ability to make sequential decisions based on its actions consequences. The agent learns through trial and error, striving to maximize a cumulative rewards signal, which serves as its guiding principle. This dynamic field of study, with applications ranging from robotics and gaming to autonomous system and recommendations engines, focuses on the concept of optimal decision making under uncertainty, and it has played a pivotal role in paving the way for machines that can adapt and optimize their behaviour in complex, real world scenarios. As reinforcement learning continues to evolve, it holds immense promise for the development of intelligent, autonomous system capable of making decision and learning in environments where clear instruction or supervision is often limited.
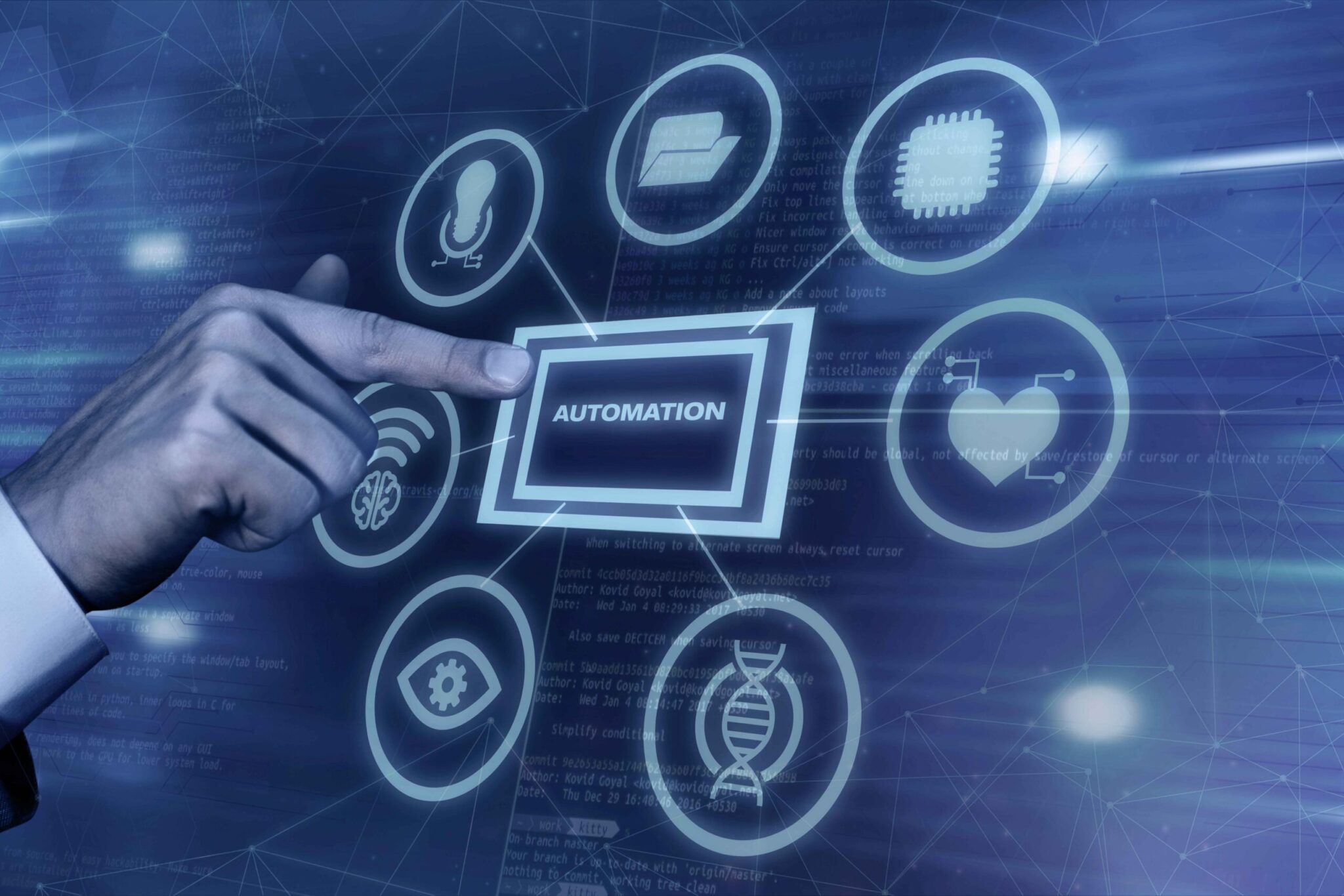
Applications of Machine Learning
Machine Learning has found application across a multitude of domains. Here are some key areas where it has made a significant impact:
- Healthcare: Machine Learning is used for diagnosis diseases, predicting patients outcomes, and drug discovery. Image recognition and natural language processing (NLP) help in interpreting medical images and texts.
- Finance: Machine Learning is used for fraud detection, algorithmic trading, credit scoring, and risk management. Predictive models assist in making data-driven investment decisions.
- Autonomous Vehicles: Self-driving cars are relying on machine learning to navigate roads and make real time decision based on sensor inputs, such as lidar, radar, and cameras.
- Natural Language Processing (NLP): Natural Language Processing (NLP) enables machines to understand, generate, and interact with human language. Chatbots, language translation, and sentiment analysis are a few examples.
- E-Commerce and Recommendation Systems: Websites like Amazons and Netflix employ recommendation system that use ML to suggest products or content tailored to a user’s preferences.
- Manufacturing: ML helps optimize production processes, reduce defects, and predicts maintenance needs through predictive maintenance.
- Marketing: Marketers use machine learning to personalize advertising campaigns, analyze customer behaviour, and forecast market trends.
- Image and Video Analysis: ML can recognize objects, faces, and gestures in images and videos. This has applications in security, entertainment, and social media.
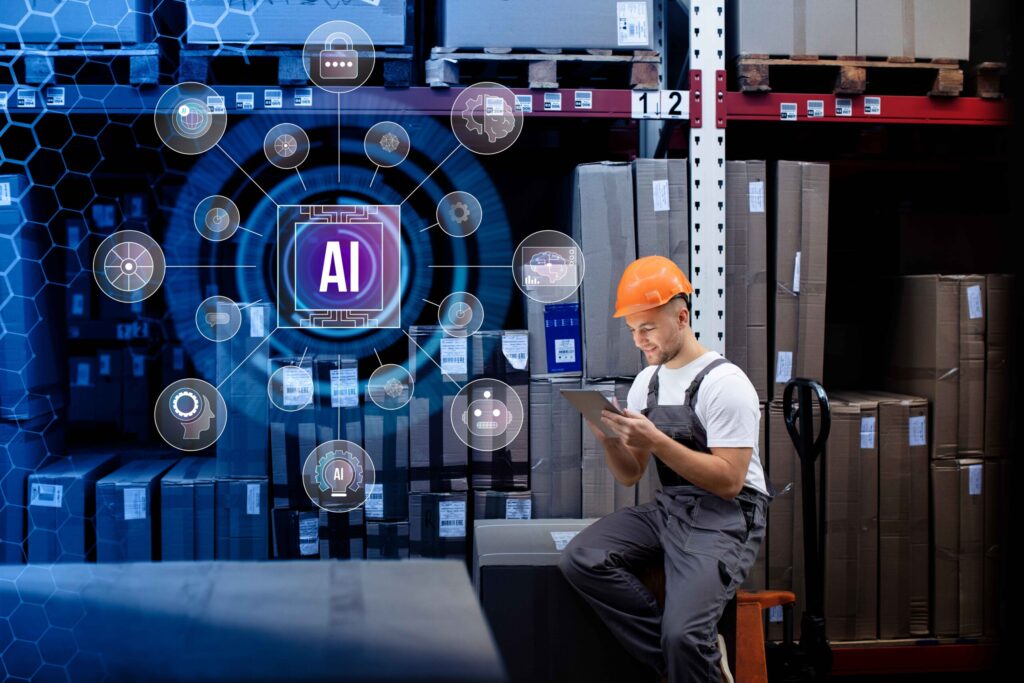
Advancement in Machine Learning
Machine Learning is an ever-evolving field with constant advancements. Some notable advancements include:
- Deep Learning: Deep Learning, is a subset of machine learning has gained popularity due to its success in neural networks. Deep neural networks, particularly convolutional neural networks (CNNs) and recurrent neural networks (RNNs) have improved image and texts processing.
- Transfer Learning: This approach allow models trained on one tasks to be adapted to another with minimal retraining, saving time and resources.
- Explainable AI (XAI): Researchers are working on making AI models more interpretable and transparent, addressing the “black box” problem of deep learning.
- Generative Adversarial Networks (GANs): GANs have revolutionize image generation and manipulation. They consist of two neural networks, a generator and a discriminator, competing to create realistic content.
- Federated Learning: Federated learning enables models training on decentralized data sources, preserving privacy and security.
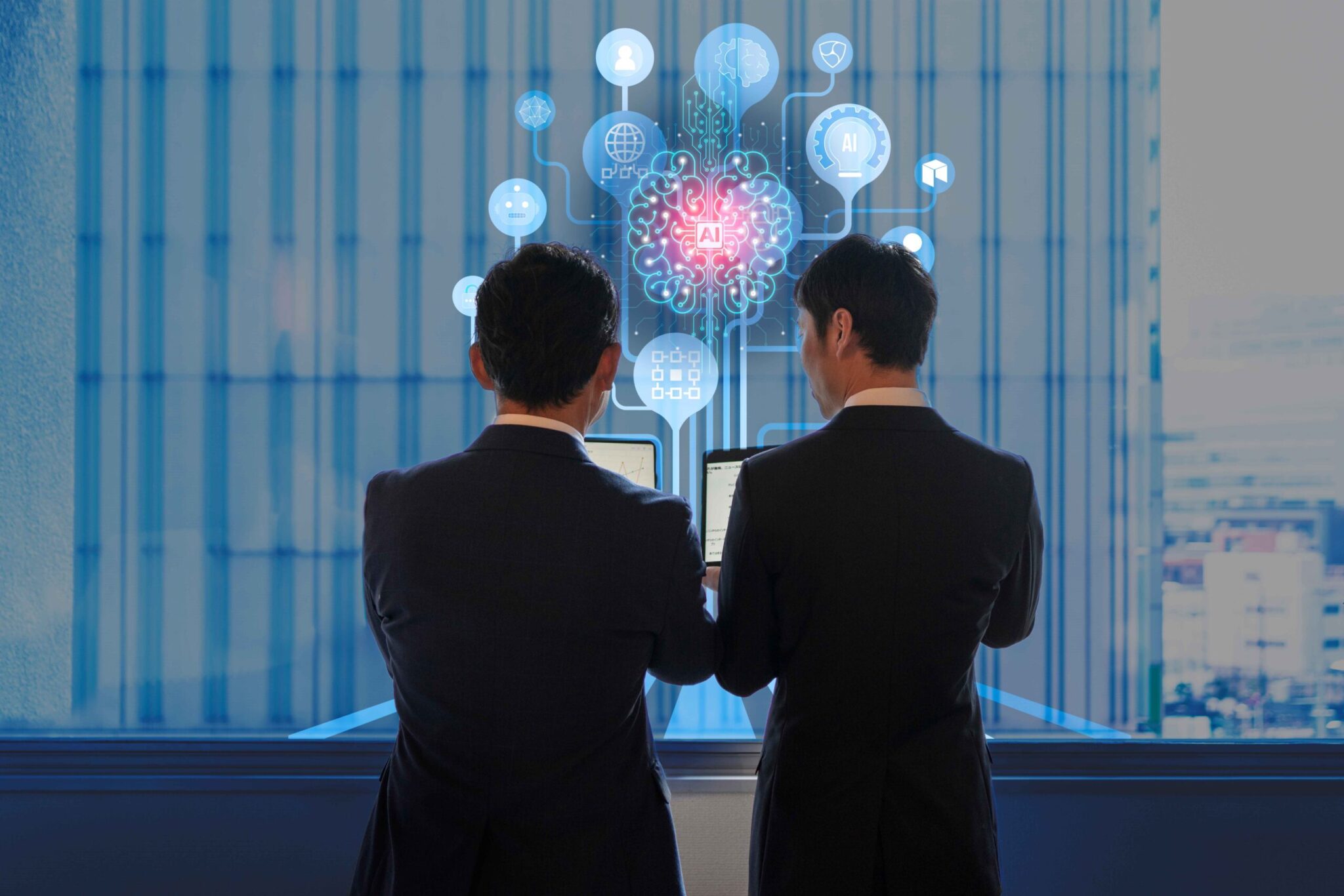
Social Impact
The impact of machine learning on society is profound and multifaceted. While it brings immense benefits, it also rise ethical and social challenges. Here are some aspects to consider:
- Ethical Concerns: ML algorithms can perpetuate bias and discrimination if not carefully designed. Fairness, transparency, and accountability in AI are crucial issues.
- Privacy: The vast amount of data is used for training machine learning models raise privacy concerns. Balancing data utility with individual privacy is a challenge.
- Job Disruption: Automation of tasks through machine learning can lead to job displacement in some industries, emphasizing the need for reskilling and upskilling.
- Security: ML system can be vulnerable to adversarial attacks and misuse, and securing AI models is a grown concern.